Beside Head of LIMM, Pham Kim Ngoc, the investigative team of this project is from the Research Group of Center for INOMAR, VNU-HCM and University of Science, VNU-Hanoi.
1. Introduction
Synapses play a crucial role in transmitting neural signals, and synaptic plasticity enables changes in synaptic strength, which allows the brain to learn from experiences. With the rapid progress in neuromorphic electronics, significant efforts have been made to design and create electronic devices that replicate synaptic functions. This increasing interest in the field is paving the way for innovative hardware architectures for artificial intelligence. This research focuses on the research of memristor and RRAM artificial synapses utilizing two-dimensional (2D), Metal-organic framework materials, which are controlled by electrical, optical, and mechanical stimuli.
2. Demand for electronic Synapse devices
Traditional Von-Neumann computing architectures are currently facing challenges due to limitations in speed and efficiency. This architecture, which relies on the physical separation of the central processing unit and memory, experiences a bottleneck due to the differing processing speeds of these units. To address these limitations, neuromorphic computing, a rapidly evolving paradigm inspired by the structure and functions of the biological brain, has emerged. In our brain, neural information processing is facilitated by the firing of spikes between neurons and synapses, enabling fast and energy-efficient operations. Neuromorphic computing aims to achieve parallel signal processing, high power efficiency, and rapid complex task computation by mimicking these biological processes.

In this context, electronic synapse devices are critical components of neuromorphic computing. Given the need for high synaptic density, using multiple transistors and capacitors to emulate a single synapse is inefficient.
3. Synaptic behaviours
Neuromorphic computing for learning and information processing leverages the plasticity of neural synapses in the human brain's neural network. Each synapse consists of a presynaptic terminal, a postsynaptic terminal, and the synaptic cleft, the narrow gap between the two terminals. The synapse is the functional connection between neurons and is essential for information transmission. When a presynaptic action potential arrives at a synapse, the voltage-gated calcium (Ca2+) channels open. Ca2+ ions enter the presynaptic membrane, triggering the release of neurotransmitter-containing vesicles. These neurotransmitters are released into the synaptic cleft and bind to receptors on the postsynaptic membrane, which causes Na+ channels on the postsynaptic membrane to open or close. This change alters the synaptic conductivity, corresponding to the excitatory or inhibitory postsynaptic currents (EPSC/IPSC). This process, known as synaptic plasticity, is crucial for information transmission in the human neural network.
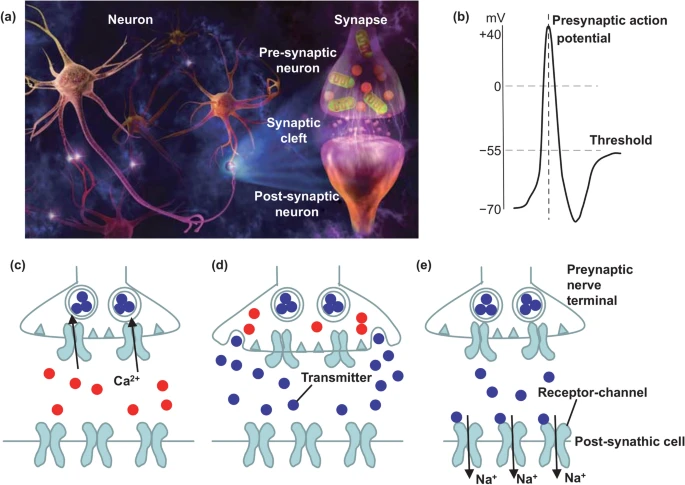
4. Spike-timing-dependent plasticity (STDP) behaviour
Synaptic plasticity, described by Hebbian theory, is the experience-dependent change in neuronal connection strength and varies with external pulse shapes. Types include short-term potentiation/depression (STP/STD) like paired-pulse facilitation/depression (PPF/PPD) and spike-number-dependent plasticity (SNDP), long-term potentiation/depression (LTP/LTD), spike-rate-dependent plasticity (SRDP), and spike-timing-dependent plasticity (STDP). STP/STD is associated with external signal recognition and is easily forgotten, while LTP/LTD involves structural changes for long-term memory. Continuous stimulation transitions short-term changes to long-term, enabling memory formation, with varying spike amplitude inducing spike-amplitude-dependent plasticity (SADP). SRDP, essential for cognitive functions, results from different pulse frequencies, and STDP, based on pulse timing at synaptic terminals, alters synaptic weight, playing a key role in information coding, learning, and memory.
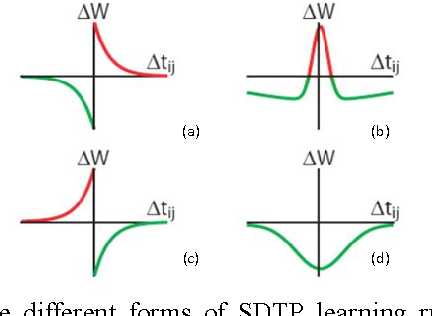
5. Memristor and its technology for artificial synapse
Different synaptic devices can be classified according to various standards. Based on the working mechanism, synaptic devices are categorized into ion migration (electrical pulses), ferroelectric (electrical pulses), phase change (including electrical and optical pulses), charge capture and release (including electrical and optical pulses), and redox mechanisms. These devices utilize materials such as metal oxides, semiconductors, phase-change materials, and perovskites.
Synaptic devices have mimicked biological synaptic functions, such as STP/STD, LTP/LTD, SRDP, and STDP. These functions have shown many application areas, which mainly include pattern recognition, logical operations, associate learning, filtering, and so on.
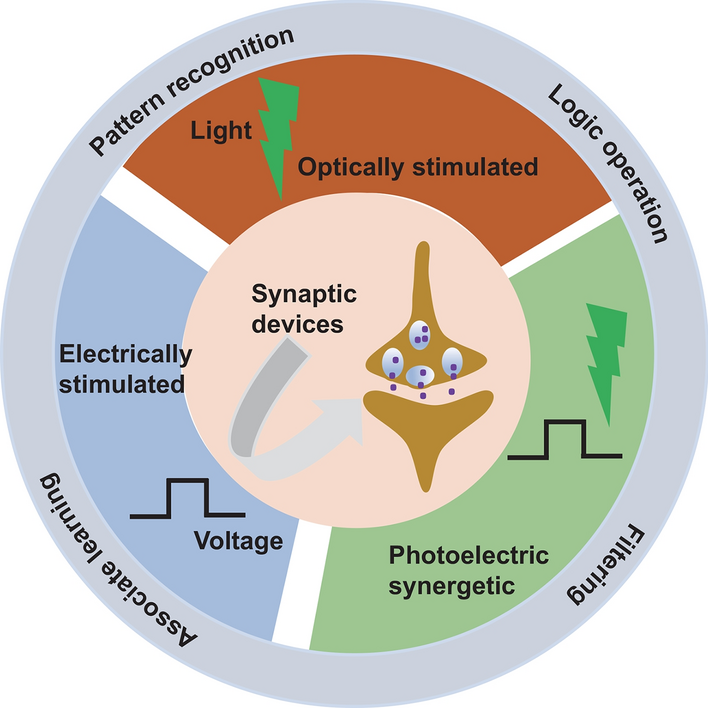
6. Memristor and its technology for artificial synapse
Structurally, synaptic devices are divided into two-terminal and transistor-type synaptic devices. The electrode port in these devices can function as a neuron connected to a synapse or as a port for signal stimulation. Two-terminal synaptic devices are easy to integrate, whereas transistor-type devices, though harder to integrate, allow for better regulation of electrical behaviours through gate voltages.
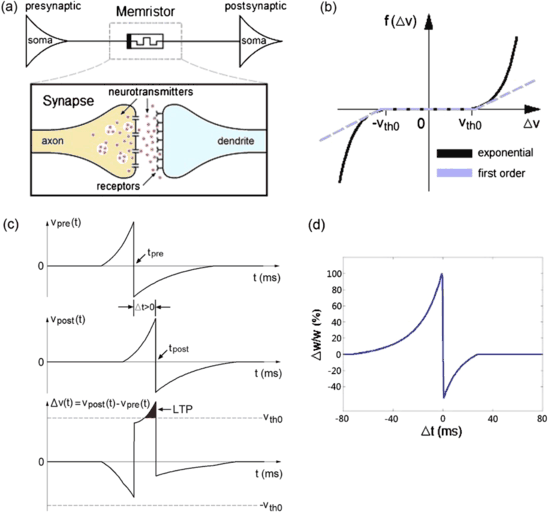
The idea of using memristors in neuromorphic computing stems from their ability to mimic the behaviour of synapses in biological neural networks. In biological synapses, the connection strength between neurons changes over time through synaptic plasticity. Similarly, memristors can adjust their resistance based on the history of electrical charge passing through them, mirroring synaptic plasticity in biological systems. Implementing memristors as synapses in artificial neural networks (ANNs) offers several advantages over traditional digital neural network implementations.
• ANNs need multiple resistance states, making transistors inefficient. Memristors like RRAM and PCM achieve this with analogue and multilevel resistance switching.
• Memristors synchronize memory and computation, overcoming the von Neumann bottleneck. They regulate resistance in response to voltage signals, allowing direct use of memristors as synapses, eliminating the need to move data to separate memory locations.
7. Perspectives
Plenty of functional materials have been used to fabricate synaptic devices in recent years. These synaptic devices have various performance indicators, such as pulse width, device size, energy consumption, completeness of synaptic functions achieved, dynamic range, the number of states, linearity of weight change, retention, and endurance. Each of these parameters should meet their corresponding requirements as synapses in neural networks for efficient neuromorphic computing.
Journal Articles
Conference Articles and Poster
Post a Comment